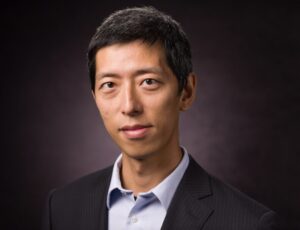
Cheng Wang
Title(s):
Assistant Professor
Office
Durham 335
613 Morrill Rd
Ames, IA 50011
Information
Education
- B.S., Peking University, 2009
- Ph.D. Physics, The University of Texas at Austin, 2015
Experience
- Research Scientist, Center for Brain-Inspired Computing (C-BRIC), Purdue University, 2019-2022
- Senior/Staff Engineer, Storage Mediua Research Center, Seagate Technology, 2016-2019
- Electronic Design Engineering Intern, Seagate Technology, 2015-2016
- Process Design Engineering Intern, Western Digital, 2013 summer
- R&D Intern, Schlumberger Research, 2012 summer
Research
Machine Learning (ML) Hardware Acceleration (with beyond-CMOS technologies)
Providing efficient computational systems to meet the growing demands from processing machine learning (ML) tasks presents exciting opportunities for research across the computational stack from Hardware to Algorithm. I would like to investigate how to enable energy-efficient and robust artificial intelligence (AI) through a co-design approach involving device, circuit, architecture, and algorithm.
Major thrusts include but not limited to: Device-architecture-algorithm co-optimization of in-memory computing systems Integration and optimization of emerging memory technologies for application-specific architecture (such as systolic arrays) Hardware-aware network architecture search and pruning
Neurmorphic Computing and Brain-Inspired Computational Models
At present, researchers in ML and neuromorphic communities are striving to meet the challenge of building intelligent electronic hardware that can reach brain-like cognitive abilities with a similar level of efficiency and robustness. I am interested in gaining inspirations from the biological systems towards developing better systems for processing complex cognitive tasks. While deep neural networks have demonstrated remarkable success, I am interested in exploring various computational models that may achieve improvements in efficiency, robustness, and explainability. Since conventional CMOS gates are not designed for such types of AI workloads, I will explore hardware implementations of neuromorphic computing with diverse device/material technologies. Major thrusts include but not limited to: Developing efficient and robust spiking neural networks for various AI tasks (vision and language) Exploration of unconventional computational models such as oscillatory activation and stochastic computing Leveraging emerging device physics to provide highly efficient neuromorphic functionality
Nanoelectronics and Spintronics for Efficient AI
As AI model size and amount of data being processed grow exponentially over the recent years, the urge of developing efficient AI is stronger than ever.nThe exciting development in beyond-CMOS devices and materials provide us a gold mine for prototyping novel AI hardware. However, it remains to be seen that if any of the novel technologies can deliver the next giant leap in hardware technology. Meanwhile, the rich dynamics in various nano-scale systems may also give us inspirations for representing and processing information. Interested directions: Searching for new mechanisms in nanoelectronic and nonmagnetic devices for efficient building blocks in AI computation Enabling cross fertilization between hardware technologies and intelligent algorithms.
Publications
- Dong Eun Kim, Aayush Ankit, Cheng Wang, and Kaushik Roy, “SAMBA: Sparsity Aware In-Memory Computing Based Machine Learning Accelerator”, IEEE Transactions on Computers (2023)
- Haensch, Wilfried, Anand Raghunathan, Kaushik Roy, Bhaswar Chakrabart, Charudatta M. Phatak, Cheng Wang, and Supratik Guha.”Compute in-Memory with Non-Volatile Elements for Neural Networks: A review from a Co-Design Perspective“, Applied Materials (2023).
- Gobinda Saha, Cheng Wang, and Kaushik Roy, “Invited: A Cross-layer Approach to Cognitive Computing“, IEEE/ACM Design Automation Conference (2022).
- Kang He, Indranil Chakraborty, Cheng Wang and Kaushik Roy, “Design Space and Memory Technology Co-exploration for In-Memory Computing Based Machine Learning Accelerators“, IEEE/ACM International Conference on Computer Aided Design (2022).
- Cheng Wang, Chankyu Lee, and Kaushik Roy, “Noise resilient leaky integrate-fire neurons based on multi-domain spintronic devices”, Scientific Reports 12 (1), 1-11 (2022).
- Bing Han, Cheng Wang, and Kaushik Roy, “Oscillatory-Fourier Neural Network: A Compact and Efficient Architecture for Sequential Processing”, Conference on Artificial Intelligence (AAAI 2022)
- Tanvi Sharma, Cheng Wang, Amogh Agrawal, and Kaushik Roy, “Enabling Robust SOT-MTJ Crossbars for Machine Learning using Sparsity-Aware Device-Circuit Co-design”. 2021 IEEE/ACM International Symposium on Low Power Electronics and Design (ISLPED).
- Hussam Amrouch, Jian-Jia Chen, Kaushik Roy, Yuan Xie, Mikail Yayla, Indranil Chakraborty, Cheng Wang, Fengbin Tu, Wenqin Huangfu, and Ling Liang, “Brain-Inspired Computing: Adventure from Beyond CMOS Technologies to Beyond von Neumann Architectures”, IEEE/ACM International Conference on Computer-Aided Design (ICCAD) 2021.
- Amogh Agrawal, Cheng Wang, Tanvi Sharma, and Kaushik Roy, “Magnetoresistive Circuits and Systems: Embedded Non-Volatile Memory to Crossbar Arrays”, IEEE Transactions on Circuits and Systems I. 68, 6 (2021) Selected as Highlight of 2021 June Issue.
- Cheng Wang, Amogh Agrawal, Eunseon Yu, and Kaushik Roy, “Multi-Level Neuromorphic Devices Built on Emerging Ferroic Materials: A Review”, Frontiers in Neurosciences 15: 661667 (2021).
- Morgan Williamson, Cheng Wang, Pin-Wei Huang, Ganping Ju, and Maxim Tsoi, “Large and Local Magnetoresistance in a State-of-the-Art Perpendicular Magnetic Medium”, Nanotechnology, Science and Applications 14, 1-6 (2021).
- Cheng Wang, Pin-Wei Huang, Ganping Ju, and Kuo-Hsing Hwang, “Exchange Coupled Composites” (for memristive synapses), US Patent Application 16/255,698 (2019).
Primary Strategic Research Area
Secure Cyberspace & Autonomy