Doug Jacobson’s leadership in cyber security research and education began before high-speed internet, smartphones and Google. It even predates the term “cyber security” itself. Jacobson, Stanley Chair in Interdisciplinary Engineering, University Professor of electrical and computer engineering, and director of the Center for Cybersecurity Innovation and Outreach, was one of the few who saw “information … Continue reading Writing the Script
Category: Uncategorized
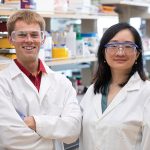
Powerhouse of the cell, key to new treatments: Mitochondrial DNA manipulation offers insights into related diseases
If you remember just one thing from high-school biology, it’s that the mitochondria are the powerhouses of the cell. But could mitochondria also hold the key to gene therapy and new tailored drug development for diseases and disorders? Zengyi Shao, Vernon Guse Faculty Fellow and associate professor of chemical and biological engineering, and her team … Continue reading Powerhouse of the cell, key to new treatments: Mitochondrial DNA manipulation offers insights into related diseases
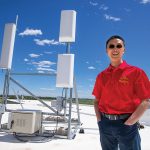
Wireless Living Lab: At-scale, real-world broadband testbed for connected rural communities
Iowa State is building a wireless “living lab” in central Iowa, laying the foundation for more affordable rural broadband service. The project will create a research testbed for a wide range of wireless technologies across Iowa State’s campus, the city of Ames and surrounding farms and rural communities in central Iowa – with an application … Continue reading Wireless Living Lab: At-scale, real-world broadband testbed for connected rural communities
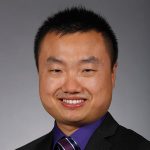
Tapping smart meters’ potential for grid resilience
Problem: Enhanced electric grid monitoring is needed to promote renewable integration while ensuring reliability, but current approaches rely on expensive sensors. Smart meters that electric utilities are already using for billing have potential, but without new computing innovations, smart meters only can provide limited insights into grid performance. Project: Wang will unlock the potential of … Continue reading Tapping smart meters’ potential for grid resilience
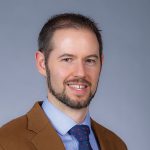
Biomanufacturing better treatments
Problem: Therapeutic cells show promise as a new treatment option for chronic illness, but advances must be made in manufacturing reproducibility to get therapeutic cells out of clinical trials and into widespread use. Project: Reuel will develop novel, real-time sensors and reinforcement learned (RL), dynamic control policies to improve reproducibility in large-scale manufacturing of differentiated, … Continue reading Biomanufacturing better treatments